With every passing day, Artificial Intelligence (AI) is being integrated into our more and more. From as small as scrolling through Instagram reels or YouTube shorts to running the biggest of businesses, and luring customers to buy products, AI is everywhere. It affects our decisions in almost every step of any process. With the advent of ChatGPT and other LLMs, We are now even flooded with AI-generated content, including ultra-realistic deepfakes. As many as the benefits of AI may be, one of the biggest limitations right now is not supporting the content generated with relevant reasoning which is a huge thing to consider in the decision-making process. AI might even generate false information which, without relevant reasoning, would need to be fact-checked or thought through manually. This limits the ability of AI to save time and effort and in some cases might even increase them. So, To overcome this, We have a new type of AI, Explainable AI (XAI).
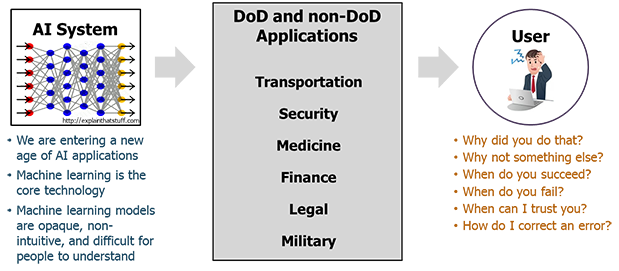
Explainable AI
Explainable AI or XAI is a new area of study in the field of Artificial Intelligence that is focused on making AI output more understandable for humans (new to AI? Check this post). It’s a set of tools, methods, and techniques that help humans interpret the decision-making of a machine-learning model while keeping the highest level of accuracy for predictions (To learn more about AI vs. Human Intelligence, Read here).
By shedding light on the workings of AI models, We can enhance user trust as well as identify and fix any bugs or biases originating in them. This additional layer of transparency makes sure that AI is not only accurate but also ethical, reliable, and fair. Users can be more confident in the outputs generated by AI which in turn would lead to better decision making. Hence, This also strengthens the bond between humans and AI facilitating safe and responsible integration of AI applications in our daily lives.
Explainable AI principles
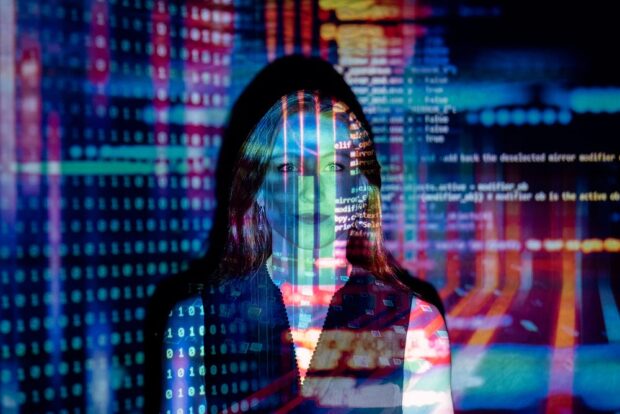
Naturally, Explainable AI is built on a set of principles that enhances transparency, accountability, and trust with its users. Some of these principles are:
- Transparency: one of the main principles of Explainable AI. Every step in the decision-making process should have clear and concise reasoning supported by the AI model.
- Interpretability: AI models should not only be transparent but interpretable as well. Users should be able to get logical explanations to understand the behind-the-scenes of a decision, giving answers to ‘Why’ or ‘How’ questions.
- Accountability: XAI models should be responsible for their decisions. This ensures that any biases, bugs, or inaccuracies are minimized, thereby, increasing the trust in its outputs.
- Fairness: XAI models should be fair and just. This leads to ethical and responsible decisions, increasing its trustworthiness.
- User-Centric Design: As with any system, XAI systems should be built with the user in mind. They should be able to give explanations good enough for users to understand them logically.
By following these principles, We can make sure the Explainable AI (XAI) systems will be able to build a bond of trust with its users, building a better understanding and integration of AI in our daily lives. To learn more about Explainable AI principles, Check out this post.
Explainable AI Examples
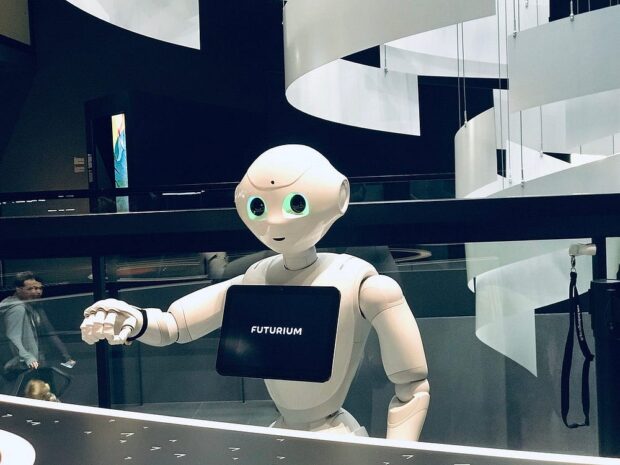
- Healthcare: We can use XAI to speed up diagnosis and image analysis. It can lead to transparency and understandability in the decision-making for patient care.
- Financial Services: XAI can make the loan and credit approval process more transparent. It can speed up credit score calculation, Wealth, and risk management. It can help solve customer complaints with valid reasoning, maintaining transparency and trust.
- Legal: XAI can accelerate prediction and risk assessment. It can increase resolutions by providing details DNA analysis, prison population analysis, and crime forecasting.
- Customer Service: XAI is used in chatbots to directly interact with customers by giving relevant information and answering questions with proper reasoning, keeping bonds strong with customers.
Tools for Implementing Explainable AI
Explainable AI (XAI) has been in the market for quite some time. Therefore, There are a variety of tools available to facilitate AI models backing up their decisions with relevant information for the user. Some of the noteworthy and important tools are:
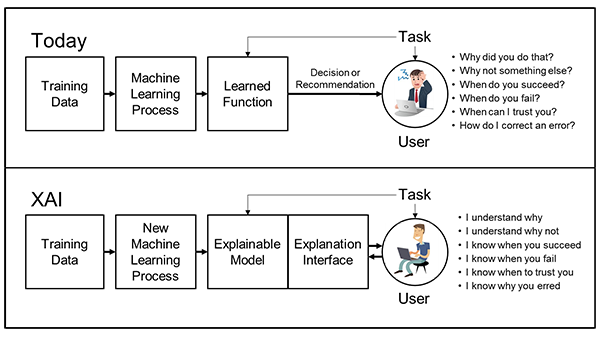
- LIME (Local Interpretable Model-Agnostic Explanations)
LIME is one of the most popular tools to provide explanations behind any machine learning model. Its name highlights a summary of how it works. ‘Local’ means its explanation is based on the current input. It highlights the parts of current input that influenced its decision. ‘Interpretable’ — There is no meaning of explanation if it can’t be interpreted or understood by a human. ‘Model-Agnostic’ — It doesn’t ‘peek’ into the model and is still able to provide an explanation.
- SHAP (SHapley Additive exPlanations)
SHAP uses game theory to explain a machine learning model decision. It uses Shap values or ‘Importance’ values from game theory to all the features indicating how much each contributed to the final decision.
ELI5 or ‘Explain Like I am 5’ is a Python library that aims to explain the machine learning models by telling weights, showing important features, and helping you visualize the predictions. It supports a wide variety of frameworks like Scikit, and XGBoost.
Alibi is an open-source Python library for interpreting and explaining machine learning models. It supports a wide range of methods like a black box, white box, local and global explanation methods of classification, and regression models.
A comprehensive open-source toolkit by IBM providing algorithms, tools, and resources for developers to better develop interpretable and understandable AI models.
To sum up, These tools are strengthing the base to make Explainable AI (AI) to make AI more understandable, explainable, trustworthy, and transparent. It also empowers data scientists, students, businesses, and stakeholders to speed up processes and make better decisions. For example, LIME creates a surrogate model that builds upon the original model’s predictions. SHAP provides the importance of each feature in the prediction. ELI5 provides visualization of these features. Alibi offers algorithms to interpret models. IBM AI Explainability 360 serves as a guide to creating XAI.
Challenges in Explainable AI and potential solutions
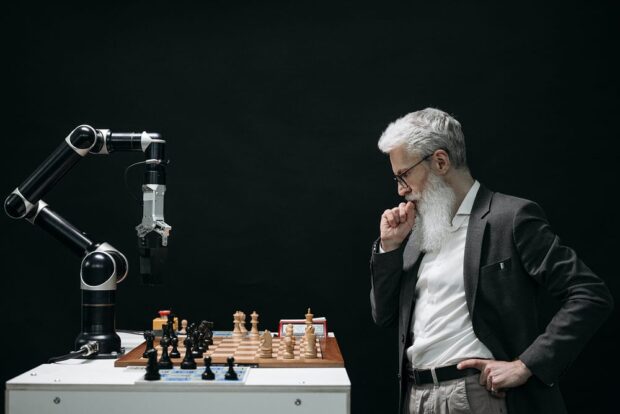
Even with all the advancements, There are still challenges to be dealt with in the field of XAI:
- Complexity in Interpretation: The increasing complexity of AI models leads to complex models that are difficult to interpret. We can address this change by improving our visualization tools to provide better insights into output.
- A trade-off between Accuracy and Explainability: A smaller model is less accurate and very explainable. A larger model is more accurate but less explainable. We need to prioritize and create a balance between the two depending on the use case of a model.
- Lack of Standardization: Currently, We don’t have any universally accepted standards to measure the explainable quality of an AI. We need to set standard metrics to compare the AI models.
- Privacy Concerns: XAI, like current AI, can potentially reveal sensitive data. To overcome this issue, We need better testing techniques and developers need to implement algorithms to stop privacy leaks.
To learn more about explainable AI challenges, Read here.
The Future of Explainable AI
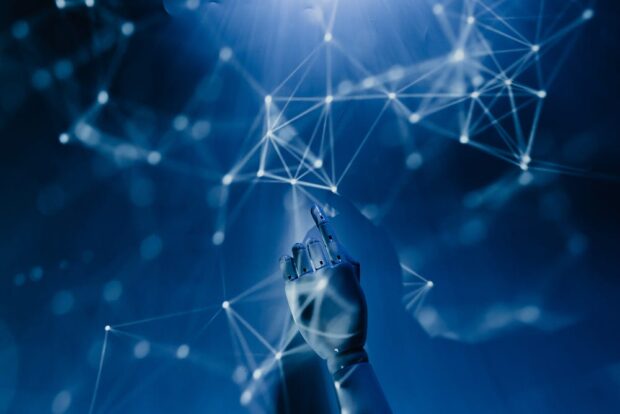
The advancements of XAI will be an integral part of the future of AI for several reasons:
- Transparency and Trust: As AI becomes increasingly complex, It will be important more than ever to provide proper explanations for its decisions. So, XAI will play an essential role in making AI systems more reliable and trustworthy.
- Regulatory Compliance: As AI progresses, There will be increasing regulatory compliance. XAI will help businesses explain that their AI models are fair, just, and compliant with these regulations.
- Improved Decision-making: XAI, at its origin, will be helping users make better decisions with proper reasoning. This will facilitate informed decisions, more efficiency, and better productivity.
Conclusion
The field of Explainable AI (XAI) is vast and ever-expanding. By uncovering the facts behind AI decisions, We can make them better understood by humans, leading to informed decisions, better efficiency, and increased productivity. As AI models continue to evolve and become increasingly complex, challenges like the AI Unlearning problem will need to be addressed, making XAI increasingly important as the business and end customers’ daily lives will be dependent on the decisions of these models. Therefore, the Importance of XAI cannot be overstated. The future of XAI is rich with promise, and as we continue to innovate and refine our tools and techniques, the full potential of Explainable AI will undoubtedly be realized.
Thanks for reading! Please follow for more articles about Artificial Intelligence!
For any of your custom AI or software development needs, Hire me as a freelancer on my personal website.